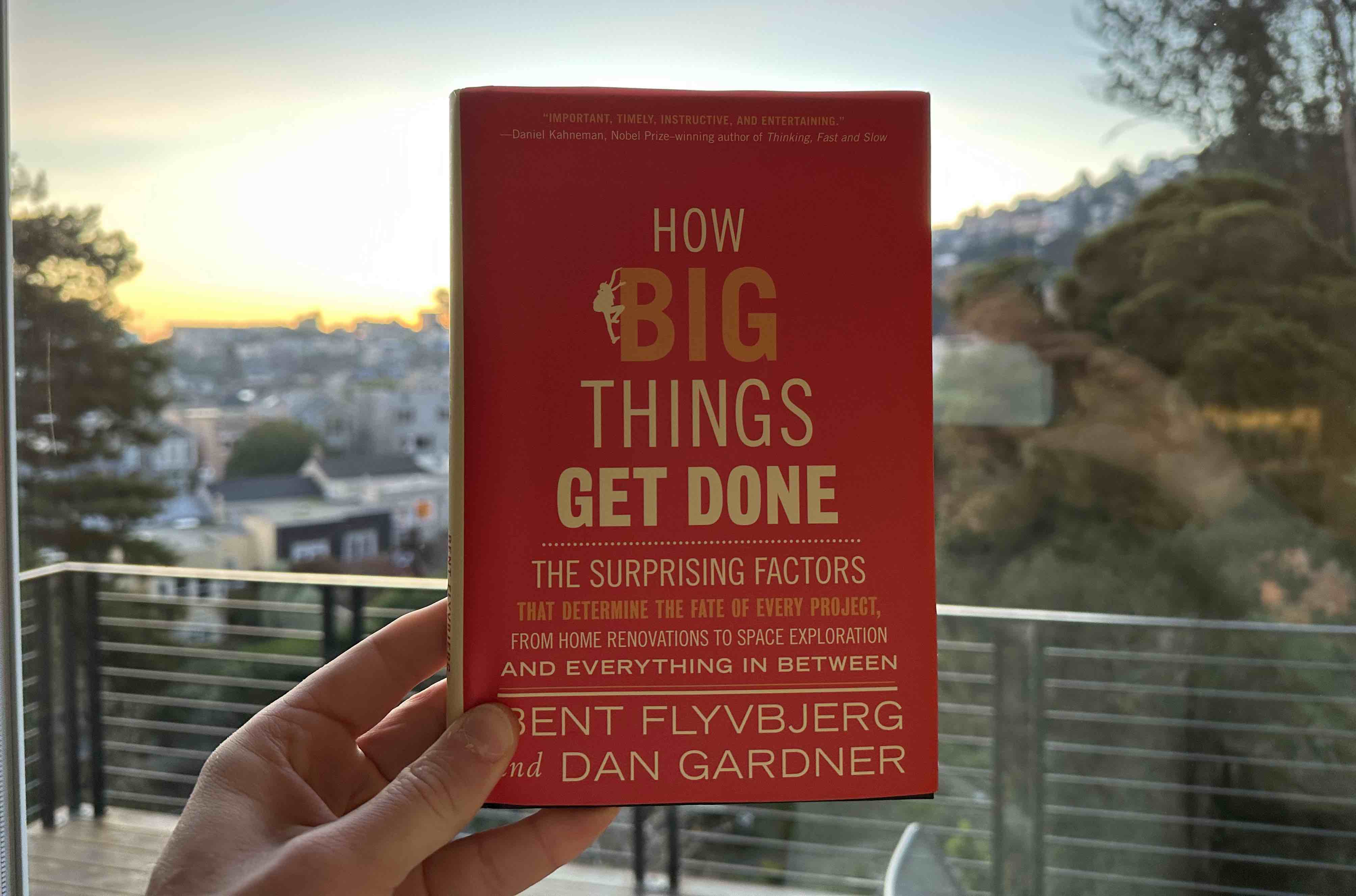
Notes on How Big Things Get Done
How Big Things Get Done by Bent Flyvbjerg and Dan Gardner is a fascinating look at why some megaprojects fail so resoundingly and why others succeed under budget and under schedule. It’s an exploration of planning methods, the role of expertise, the value of benchmarking similar projects, and much more. Not directly software engineering related, but very relevant to the work. Also, just well written.
“Think slow, act fast”
It’s fine for planning to be slow (p17), as long as delivery is fast. Each moment during delivery (the actual execution of a task) is a moment something can go wrong, so condensing that timeline is essential to reduce risk. That is of course, actually condensing the timeline, not just lying about it as discussed in the “Honest Numbers” section below.
Planning phase is preferable to delivery phase because (p18) “the costs of iteration are relatively low” during planning. The example of Pixar is used, where they storyboard films up to eight times before moving into delivery phase. This is a large investment, but it’s a much cheaper investment than making a bad film.
It’s also much easier to avoid “lock in”, which is premature commitment (p42) if you plan extensively before moving to delivery. Once you begin delivery, modifying the plan is quite challenging. To make this point, the authors make an extensive comparison between the building of the Sydney Opera House and the Bilbao Guggenheim Museum. The former changed plans frequently with massive delays, the later delivered ahead of time and under budget. (In part due to significant use of modeling for the Bilbao, discussed in “Pixar Planning” section below.)
Also a good discussion of good planning starting from the end and reasoning backwards. Have a clear sense of why you’re doing something before you try to solve it. There’s a mention of Amazon’s Press Releases (p52)–write a future internal press release as a mechanism to pitch your project–as one mechanism to support reasoning backwards.
“Pixar planning”
The book argues that good planning is “Pixar planning” (p60), where you’re able to iterate quickly and cheaply. The average Pixar film is storyboarded 8 times (p70) to cheaply explore improvements.
This means that good planning requires modeling techniques, including modeling software(p68)
as one technique, to support rapid, cheap exploration.
The example of Frank Gehry extensively modeling out his buildings in simulation software
is used to explore how he was able to deliver the Bilbao Guggenheim Museum so effectively.
(A few years ago I played around with creating the systems
library for modeling systems thinking problems, which was one of my experiments towards
this end.)
Finally, the book also observes that learning happens not only within projects but also across projects (p159). Solar and wind projects are significantly less risky than nuclear projects in part because solar and wind projects deploy hundreds or thousands modular units, rather than one very large unit. Even if some wind turbines are poorly designed or installed, they can learn from than for the next ones. Learning to build nuclear power plants is much harder, since so few of the projects occur.
Dataset of projects
One fascinating idea, mentioned a number of times but not deeply explored, is that the authors have a “database of big projects” (p4) where they track the scope and outcome of various projects. This was initially 259 projects (p111), growing up to 16,000 projects over time.
This is a remarkable resource because it makes it possible to benchmark projects against similar projects, referred to as “reference-class forecasting” (p109), or at least benchmark against something, “reference point” (p111). I’ve been thinking a lot about benchmarking recently, and this is definitely something that furthere my interest. (This book also mentions Superforecasting a handful of times, so I’ve ordered a copy of that to take notes from as well.)
Things can be inexperienced
This book says something I’ve understood for a while but never articulated clearly, which is that things can be inexperienced, such like people can (p86). They use the example of a potato peeler that cuts your fingers when you use it, which you replace with iterations of better potatoe peelers than are less likely to cut your fingers. The final edition is an experienced thing, whose design incorporates significant learning into it.
You could probably write an entire book on just that idea alone. Perhaps combined with the observation that we often lose sight of why things work. Perhaps that book is The Design of Everyday Things.
“Honest Numbers”
I also appreciated the discussion of “honest numbers” (p3), which is really a discussion about dishonest numbers and how they justify many projects. A recurring theme in the book is that many leaders deliberately misinform stakeholders about potential costs in order to build commitment, reach a point of no return, and then acknowledge the full costs.
This is eloquently captured in a quote from Willie Brown (p35):
In the world of civic projects, the first budget is really just a down payment. If people knew the real cost from the start, nothing would ever be approved."
This idea, termed “strategic misrepresentation” (p26), reminds me of a poor joke I sometimes tell, which is that “Vice Presidents never miss their targets, they just move the targets to what they accomplish.” Holding the powerful to account is difficult, even if they are acting in good faith, and when they’re acting in bad faith, then it’s remarkably challenging.
This is an important issue, because often the parties who make the commitment aren’t the ones who are stuck paying it off (p38):
Drapeau got his Olypmics. And although it took more than thirty years for Montreal to pay off the mountain of debt, the onus was on the taxpayers of Montreal and Quebec. Drapeau wasn’t even voted out of office.
Incentives are hard, and harder still when there’s not possibility for accountability, as is often the case for politics.
Altogether, a quick and interesting book. Well worth a read.